3D Indoor Instance Segmentation in an Open-World NeurIPS 2023
Mohamed El Amine Boudjoghra1, Salwa K. Al Khatib1, Jean Lahoud1, Hisham Cholakkal1, Rao Muhammad Anwer1,2, Salman Khan1,3, Fahad Khan1,4
1Mohamed Bin Zayed University of Artificial Intelligence (MBZUAI) 2Aalto University 3Australian National University 4Linköping University
Abstract
We formulate the problem of 3D point cloud instance segmentation in the open world, where we relax the assumption of a closed setting of classes during the training. We propose an auto-labeling scheme to produce pseudo-labels during training and induce separation to separate known and unknown category labels. We further improve the pseudo-labels quality at inference by adjusting the unknown class probability based on the objectness score distribution. We also introduce carefully curated open-world splits leveraging realistic scenarios based on inherent object distribution, region-based indoor scene exploration and randomness aspect of open-world classes. Extensive experiments reveal the efficacy of the proposed contributions leading to promising open-world 3D instance segmentation performance.

Poster
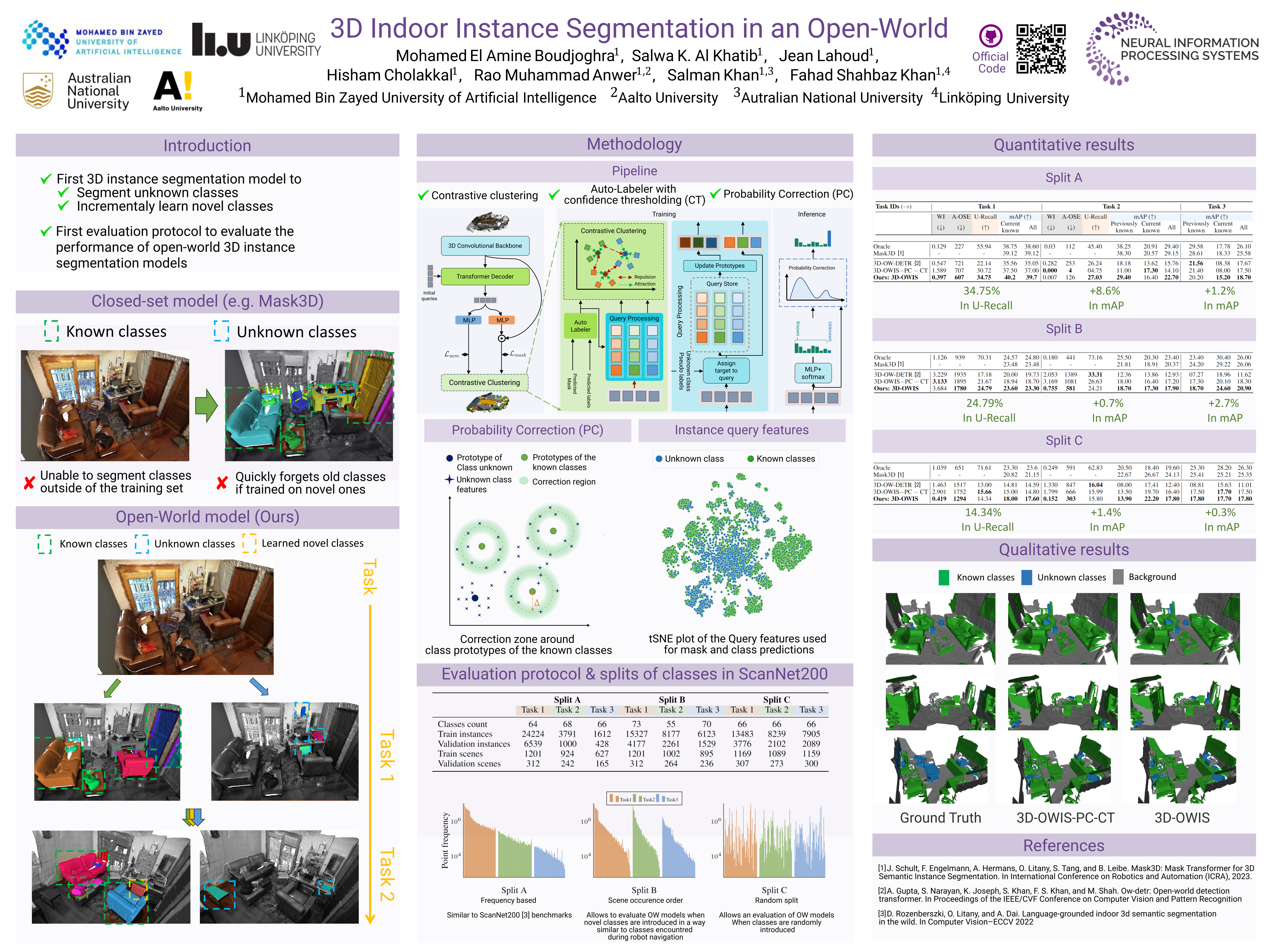
Open-world splits for 3D indoor instance segmentation
Below, we present the statistics for our ScanNet200 class splits. These splits have been carefully designed to provide a rigorous evaluation framework for open-world 3D point cloud instance segmentation models within indoor environments.
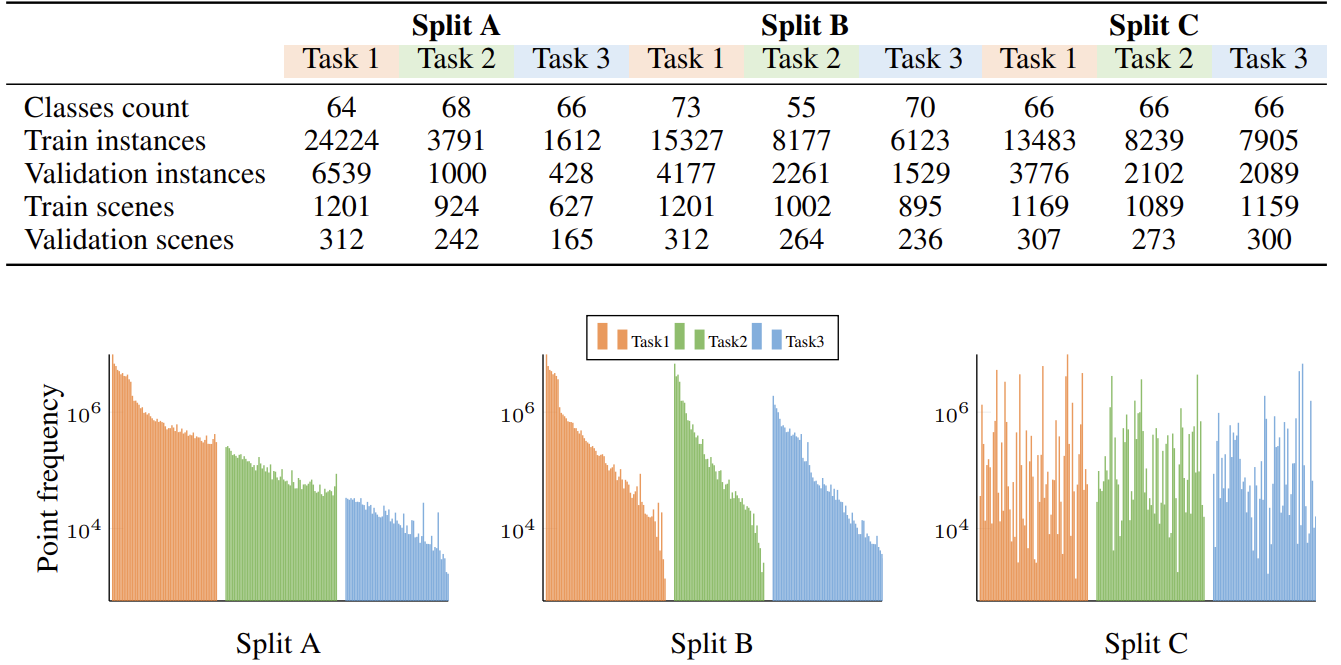
Qualitative results
Unknown classes segmentation
We show bellow the predicted known and unknown instances compared to the ground truth (Green for knowns and blue for unknowns) instances by 3D-OWIS.
Scene 1
Ground truth
Predicted known instances
Predicted unknown instances
Scene 2
Ground truth
Predicted known instances
Predicted unknown instances
Learning novel classes
We show the predictions of 3D-OWIS throughout the three tasks.
Task 1
Task 2
Task 3
BibTeX 🙏
@inproceedings{ boudjoghra2023d, title={3D Indoor Instance Segmentation in an Open-World}, author={Mohamed El Amine Boudjoghra and Salwa K. Al Khatib and Jean Lahoud and Hisham Cholakkal and Rao Muhammad Anwer and Salman Khan and Fahad Khan}, booktitle={Thirty-seventh Conference on Neural Information Processing Systems}, year={2023} }